GL and the Android Lifecycle
LPGPU2 Feedback Engine
Motivation
This study arose from an attempt to track GL resources with the LPGPU2 Profiling Tool and Feedback Engine. Every glCreate* function should have a matching glDelete* function, for example, and we would like to either confirm this or else detect its omission. The LPGPU2 Test Apps were used during the development of the resource/state tracking component of the feedback script.
When the script was completed, the LPGPU2 Feedback Engine once again revealed something previously unknown: all of the GL ReplayNative-based LPGPU2 Test Apps leak resources!
Introduction
Software applications used to have a very simple lifecycle:
- Start-up
- Run
At some point it became important to ‘clean up after yourself’ and this was extended to:
- Start-up (initialise, load, setup, etc.)
- Run (workload, task, job, etc.)
- Shutdown (finalise, save, teardown, etc.)
Many applications still follow this basic model, but the need to squeeze every ounce of performance from complex platforms running dozens of apps on hundreds of processes and threads has driven Application Lifecycle Management (ALM) to more fine-grained idioms.
Large and complex frameworks, tools and services implementing well-established patterns now enjoy widespread adoption, and even Operating Systems have moved beyond Boot -> Run -> Shutdown as they can be put into sleep, hibernate, safe modes and different levels.
ALM is particularly important for mobile devices because of the inherent power limitations. The Android operating system was designed from the ground up for mobile devices. Android exposes sophisticated ALM support through its android.app.Activity class. The simplest view of the lifecycle of an Android app is show in Figure 1.
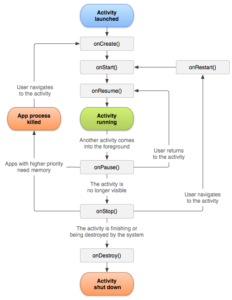
Figure 1 – Android Application Lifecycle
© Google – https://developer.android.com/guide/components/activities/activity-lifecycle.html
This lifecycle, encapsulated in the Android Activity, can be modelled as a finite state machine with seven nodes, and implemented by seven appropriately-named member functions of the class. These states and their transition states with their equivalent member functions are detailed in Table 1.
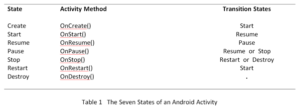
The increased functionality of better ALM comes at the price (as is so often in the case) of code complexity, because the lifetimes of all the applications resources need to be carefully shepherded through these states.
The LPGPU2 Feedback Engine has sophisticated object usage and lifetime tracking that can be used to check an application is behaving correctly throughout its lifecycle.
The Lifecycle of LPGPU2 Test Apps
Until now, we have focussed on the performance of the app main loop; the LPGPU2 Profiling Tool has been used to launch an app and begin profiling. The Tool continues profiling until the stop button is clicked. At that point no more data is gathered, the remaining profile buffer is flushed from the device, and only then is the app shut down (or killed).
It is not possible under the present framework to check for an app closing down cleanly because all LPGPU2 test apps so far, run indefinitely once inside the main loop. To rectify this, the ReplayNative app (basis of the Raymarching, Overdraw, OverdrawTex, Menger, Globe and Uber LPGPU2 Test Apps described elsewhere) has been extended to optionally run for a specified number of frames and then shut down cleanly. It is possible to run for a single frame, though because the apps are double-buffered, and because of the positioning of the swap-buffers command in the main loop, there will be no visible output in this case. It is also possible to run for zero frames in which case only initialisation and finalisation is executed – a useful test in itself.
None of this affects the LPGPU2 Profiling Tool – capable of collecting counter data even after an app crashes or if no app runs at all. The benefit here is in the collected database which will contain every single API call of an application run, from the very beginning of its lifecycle to the bitter end. This, in turn, means that the LPGPU2 Feedback Engine can analyse the profile for resource management issues accurately, in addition to the wide range of situations it already analyses, detects and diagnoses.
Insights and Improvements
This study arose from an attempt to track GL resources in the Feedback Engine. Every glCreate* function should have a matching glDelete* function, for example. Every glGen* function should also have a matching glDelete*, and we wanted to detect this. (The two glGenerate* functions, of course, are a special case and do not fit this pattern.) The LPGPU2 Test Apps were used as targets during development of the resource- and state-tracking component of the LPGPU2 feedback script.
The improved LPGPU2 Feedback Engine and the Call Sequence Analysis was run and immediately revealed something previously unknown – that all of the ReplayNative-based LPGPU2 Test Apps were leaking resources. The annotations emitted are shown in figure 2.
An investigation into this revealed an initialisation function was being called twice: triggered once by the OnCreate() method of the Activity class and again by an UpdateViewport() method that is called when the surface and framebuffers and other resources are being setup. This needs to happen when the screen is rotated, for example. The design of the ReplayNative app is such that all of the setup code was in one function. This is because ReplayNative was been based on the earlier Lua-supporting ‘Replay’ app (also described elsewhere) that supported only initialisation and rendering, not finalisation.
It seemed natural and efficient to insert a single call to the initialisation function inside the unforeseen UpdateViewport() and to just ‘walk away whistling’ safe in the knowledge that the app was not ‘designed to shutdown’, and it worked for a long time.
However, LPGPU2 Feedback Engine detected the extra initialisation calls, found no matching clean-up calls, and promptly emitted the highest level severity ‘ISSUE’ level annotations on the grounds that there is no excuse for not cleaning up after yourself. These annotations are shown in figure 2.
Orphaned sets of shader programs, shaders, vertex buffers and textures are being generated by the Carousel app as it stands. This had never been an issue before, because these apps had never been shut down cleanly under profiling.
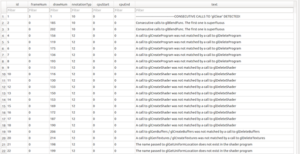
Figure 2 – Annotations emitted by the LPGPU2 Feedback Engine including many resource management issues for Shaders, Programs, Buffers and Textures
Conclusion
The LPGPU2 Profiling Tool and Feedback Engine are capable of tracking the state of resources through the entire lifecycle of an app. A design flaw in the ReplayNative base app – initially acknowledged, tactically ignored and inevitably forgotten – was unlikely to have been rediscovered without the LPGPU2 Feedback Engine.